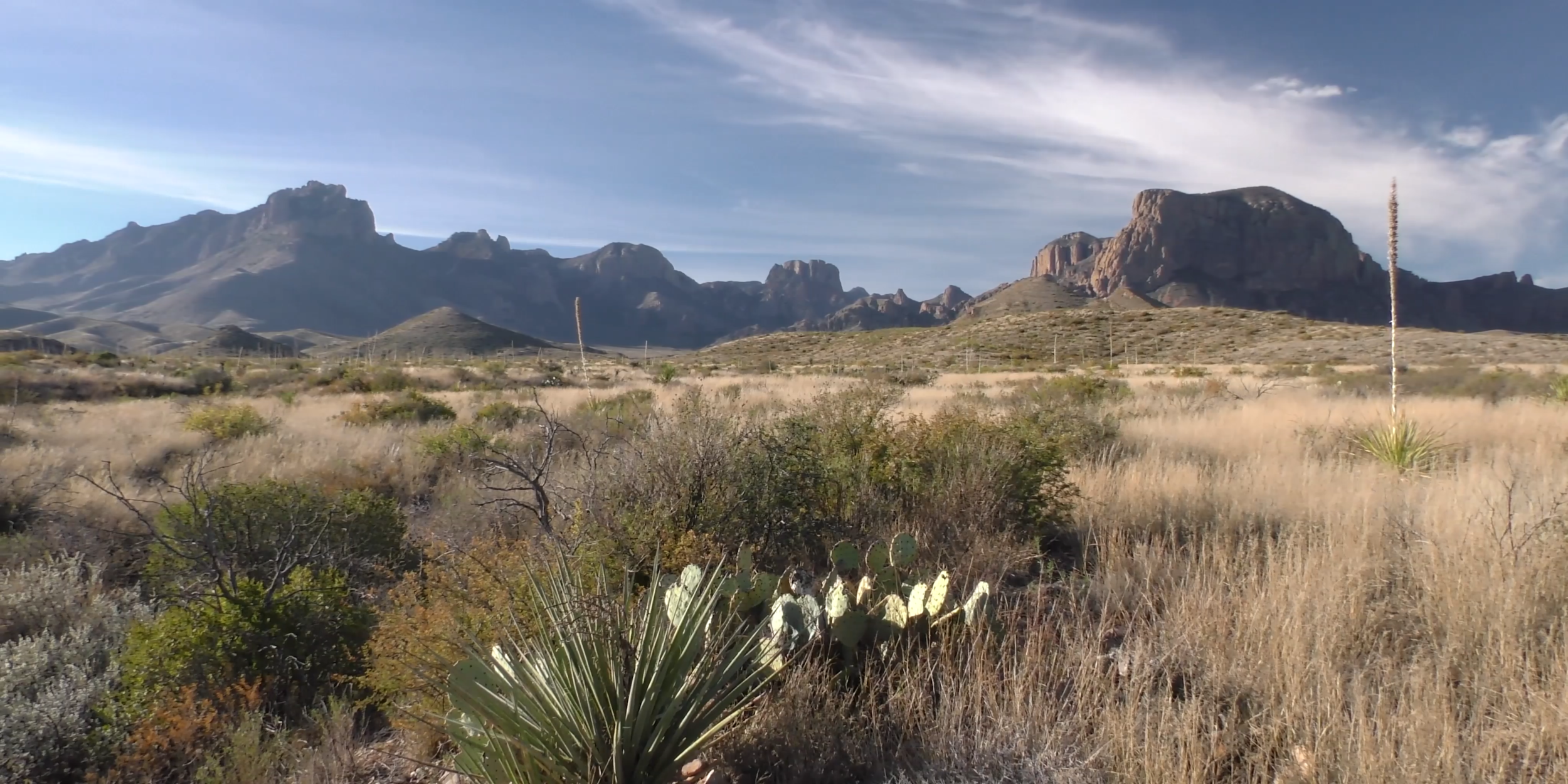
Development by Design in West Texas:
Mitigating Energy Sprawl Through Cooperative Landscape Planning
Kei Sochi, Jon Paul Pierre, Louis Harveson, Patricia Moody Harveson, David V. Iannelli,
John Karges, Billy Tarrant, Melinda Taylor, Michael H. Young and Joseph Kiesecker
May 2021
Detailed Methods: Species Habitat Suitability & Connectivity Models
By: Justin French, Dana Karelus, Carlos Gonzalez, Louis Harveson and Patricia Moody Harveson
Pronghorn Habitat Suitability and Connectivity Models
Base Data Collection
Models were generated using collar data from 379 translocated pronghorn, released in the Trans-Pecos in six translocation events over seven years. The data represent pronghorn movement over eight years. The collars were set at different fix intervals, depending on translocation event. The longest interval was three hours between fixes. The data set was subset to three-hour intervals across all collars, yielding a data set of 556,465 locations used in the model.
Habitat Suitability Model
Habitat selection and movement parameters were estimated using integrated step selection functions [55], which separate and quantify habitat selection and movement behaviors from GPS data. This results in unbiased estimates of each from a single data set.
Selection was modeled as a function of absolute elevation, slope, terrain roughness, herbaceous vegetation cover, and shrub cover. Since pronghorn occupy grasslands, and grasslands occur at mid-elevations in this region, we included a quadratic effect of elevation. This allows the model to seek an optimum, where the rate at which probability “falls off” is an estimated parameter. Edaphic variables were derived from a 30m digital elevation model (available via API through Amazon AWS https://registry.opendata.aws/terrain-tiles/). Vegetation variables were obtained from Multi-Resolution Land Characteristics Consortium, 2016 data (https://www.mrlc.gov/data). Variables were selected using AIC scores and model weights [56], which overwhelmingly supported the top model.
The resulting habitat kernel was projected across the Trans-Pecos at 30m resolution. This map represents a probability distribution of pronghorn selecting a given region of space, independent of any movement constraints.
Connectivity Model
We derived connectivity maps by simulating pronghorn movements across the habitat landscape, represented by the habitat kernel. We used the R package SiMRiV to simulate correlated random walks [57, 58], based on observed movement parameters of pronghorn. This package weights the step distribution against a map of movement costs to determine each step a simulated individual takes. We used a negative linear transformation of the habitat kernel ((1−habitat)/max(1−habitat)) to transform it to a cost surface. We generated 2000, evenly spaced locations across the Trans-Pecos and retained only those with a cost less than 0.8 as starting locations for each simulation. This was done to ensure even representation of suitable habitat throughout the region and resulted in 115-120 simulated individuals per simulation. Each simulated individual took 2,000 steps from its origin, yielding a trajectory of expected pronghorn movement. We fit a Brownian Bridge utilization distribution to each simulated individual[59] and averaged the resulting surfaces across individuals within each simulation. This yielded a surface representing the probability a pronghorn passed through each pixel for each simulation. This process was repeated 100 times and averaged over simulations to produce the expected space use of pronghorn across the Trans-Pecos.
Bighorn Habitat Suitability and Connectivity Models
Base Data Collection
Models were generated using collar data from 172 translocated bighorn, released in the Trans-Pecos in four translocation events over five years. The collars were set at different fix intervals and durations, depending on translocation event, and ultimately represent bighorn movement over six years. The longest interval was five hours between fixes. The data set was subset to five-hour intervals across all collars for animals tracked for >30 days yielding a data set of 26,364 locations used in the model.
Habitat Suitability Model
We estimated a resource selection function (RSF) using the maxlike estimator for presence only data [60]. This estimates the absolute probability a bighorn would use a pixel, given it encountered it, without subjective delineation of the availability of locations to the bighorn. Principal component analysis (PCA) was used to evaluate differences within environmental variables used in a maximum likelihood test. This facilitated a selection of characteristics that explained the majority of variability within the environmental variables. This method allows for the reduction of strongly correlated data groups and only utilizes the factors that explain the most variance and are not related to each other [61]. Selection was modeled as a function of absolute elevation, slope, terrain roughness and woody vegetation cover. The resulting RSF was projected across the Trans-Pecos at 30m resolution.
Connectivity Model
We derived connectivity maps by simulating bighorn movements across the habitat landscape, represented by the projected RSF. We used the R package SiMRiV to simulate correlated random walks [57, 58], based on observed movement parameters of bighorn. This package weights the step distribution against a map of movement costs to determine each step a simulated individual takes. We used a negative linear transformation of the habitat kernel ((1 − habitat)/max(1 − habitat)) to transform it to a cost surface. We generated 120 starting locations for each simulation by sampling probabilistically across the RSF surface. This was done to ensured even representation of the highly fragmented areas of suitable habitat throughout the region. Each simulated individual took 2000 steps from its origin, yielding a trajectory of expected bighorn movement. We fit a kernel density estimate of each simulated bighorns’ utilization distribution [62], and averaged the resulting surfaces across individuals within each simulation. This yielded a surface representing the probability a bighorn passed through each pixel for each simulation. This process was repeated 100 times and averaged over simulations to produce the expected space use of bighorn across the Trans-Pecos.
Mountain Lion Habitat Suitability and Connectivity Models
Base Data Collection
We used GPS location data from 21 mountain lions previously caught and collared by the Borderlands Research Institute from 2011-2018. All individuals were tracked for at least one month and had suitable data for analyses. Four individuals were from Big Bend National Park (two females, two males; all adults) and 17 were from the Davis Mountains (12 females, five males; seven subadults, 10 adults). Fix schedules on collars varied among lions. In the Davis Mountains, two females had collars that obtained fixes every three hours and all others had collars that obtained six locations per day. In Big Bend National Park, all collars obtained six locations per day except on Wednesdays and Saturdays when collars collected fixes hourly. On average, there were 1,408 ± 328 locations (± SE) per individual, ranging between 373 locations and 6,629 locations. Individuals were tracked over an average (± SE) of 275 ± 58 days, ranging between 87 days and 1,090 days.
Habitat Suitability Modeling
We estimated habitat suitability using resource selection functions (RSF; [63, 64]). For each individual, we fit generalized linear models with a binomial response of used/available (1/0), an additive effect of landform and HLI and assigned a large weight to available locations to reduce bias (used = 1, available = 5,000)[65].
To determine availability, we randomly selected five “available points” for each used point by an individual from within their respective 100% minimum convex polygon (MCP; [66]) plus a surrounding five km buffer. We extracted the values of our environmental covariates at the used and available locations. Our environmental covariates included a categorical raster of landforms and a categorical raster of heat load index (HLI); both layers were obtained from https://adaptwest.databasin.org/pages/adaptwest-landfacets with 100 x 100 m grid cells. The Landform raster originally included nine categories based on topographic position index (TPI), slope, and elevation [67]; however, due to low available/used locations in two of the categories, we collapsed these down to seven categories (valleys, hilltop in valley/local ridge in plain, headwaters, ridges and peaks, plains/gentle slopes, local valley in plain and steep slopes). The HLI raster included three categories based on aspect and slope: cool, neutral and warm.
We estimated the population level covariates from the individual models using bootstrapping by sampling estimates from eight individuals at a time, calculating the mean, replicating the process 1,000 times, then calculating the overall mean and lower and upper confidence intervals from the replicates. Using the population level beta estimates, we predicted the RSF across the Trans-Pecos. We scaled the values of the resulting raster to range between 0 (poor habitat suitability) and 1 (high habitat suitability). Habitat suitability modeling was performed in R statistical software [68] using base functions and the packages raster [69] and sf [70] for raster processing operations, and the package adehabitatHR [71] for creating the MCPs.
Connectivity Model
Connectivity modeling was performed using ArcGIS 10.7 and Linkage Mapper 2.0 toolbox [72]. We used circuit theory and least-cost path analysis to identify corridors connecting core habitat patches for mountain lions in West Texas [73–75]. Core habitat patches were generated based on the RSF using Gnarly Landscape Utilities 0.1 toolbox [76]. The RSF was aggregated to 1km2 to improve processing time and core patches were created using the following settings: moving window radius = 6723m (equivalent to half the average home range size of an adult female mountain lion); minimum average habitat value = 0.4; minimum habitat value per pixel = 0.1; minimum core area size = 25km.
Corridors were generated using the least-cost path between adjacent core patches. A resistance surface was created from the predicted habitat suitability values (RSF) using a C2 transformation [77] and then rescaled to 1km2:
Where h is the predicted scaled habitat suitability value and c is a constant, here equal to two, that allows for a negative exponential transformation from habitat suitability to resistance surface. In this way, we allowed for animals to have a greater probability to move through habitat that was not highly suitable, which is often the case for highly mobile animals that are dispersing [77, 78].
Corridors were truncated to a cost-weighted threshold of 200,000 with zero representing the highest connectivity. The corridor map was then overlaid with the core habitat patches (value = 0) to create a final map representing core habitat and linkages throughout West Texas.
Figure S5. Species habitat suitability and connectivity models for pronghorn (A1, A2), bighorn sheep (B1, B2) and mountain lion (C1, C2).